Human-machine Tradeoffs in Supply Chain Decisions (and the LA Port)
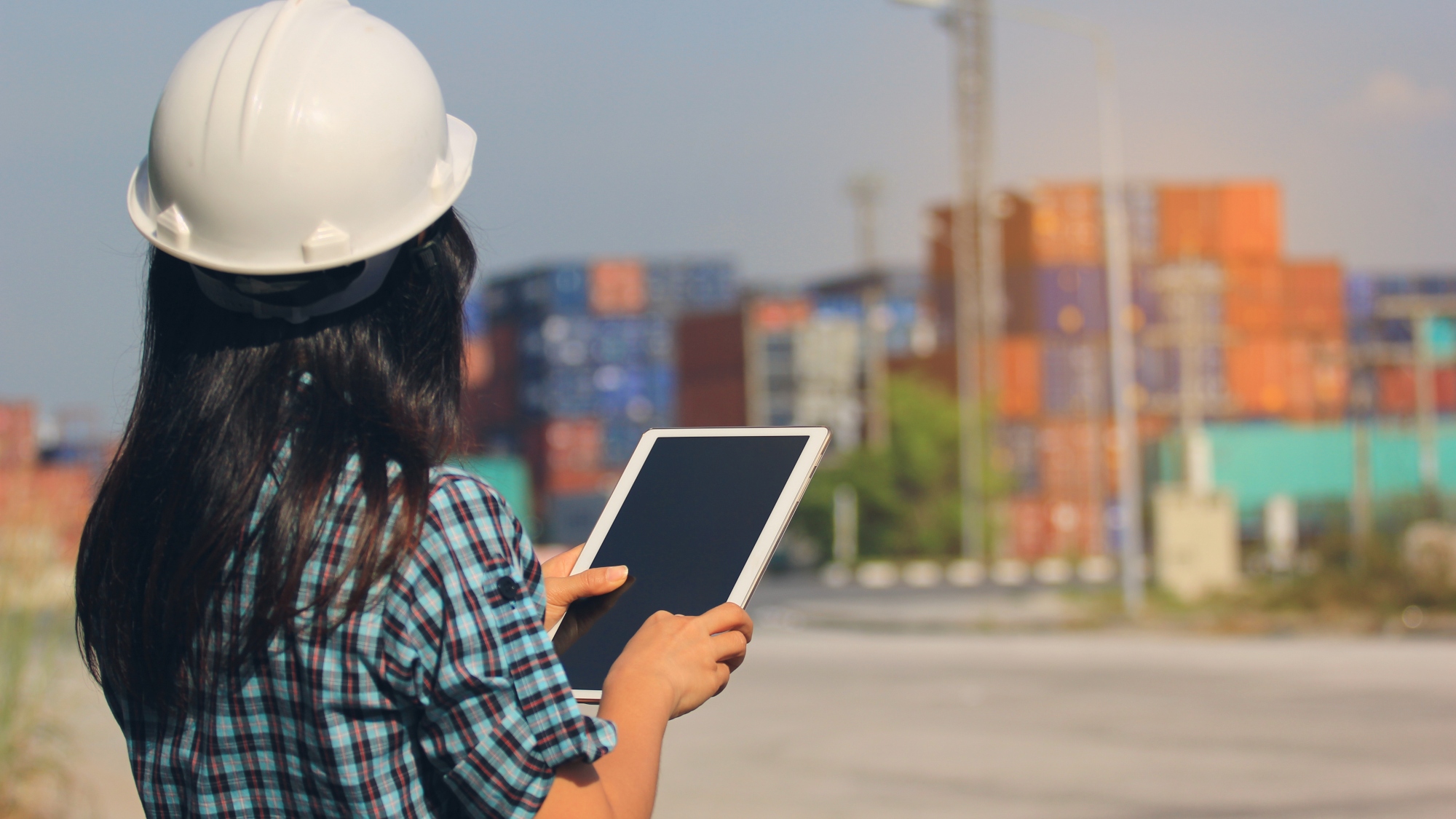
The current set of supply chain disruptions is bringing increased focus on the need for digital transformation of our supply chains. Nowhere is digital transformation needed more than the Los Angeles port, where major operational disconnects are occurring between ships, trains, and trucks. Compared to other ports like Hong Kong, Shanghai, and Rotterdam, the LA port is long overdue for a complete redesign of its digital infrastructure. (Hopefully there is SOMETHING in the Infrastructure Bill that passed this week which is dedicated to investment in this port, which is a major gateway for US imports from Asia). In particular, the need for improved integration of scheduling inbound container ships with advanced planning and scheduling of inbound trucks and trains into the terminal is one reason the LA port is only half as productive compared to other ports around the world. This is one area where machines can clearly do a better job of processing many complex activities, because the outcome (increasing the throughput of containers and limiting the wait time of drivers) is clearly expressed. However, not every supply chain and procurement decision fits neatly into this category. I believe that automation is appropriate for the “right” set of supply chain tasks, that do not require consideration of context, interpolation, or double entendre situations.
For instance, many supply chains find themselves in periods of increased volatility – both customer-facing demand variation, and supply-side shortages and capacity limitations that are impacting delivery of goods and services. The vibrations that occur between supply and demand can cause considerable swings and this can seem to be a chaotic situation. To address this issue, category managers in procurement may increase the planned lead times in an MRP reorder point system to 90 days, or may simply enter it at this face value quoted buy a supplier, without further negotiation with the supplier. This could cause inflated planning lead times to infiltrate a planning system, because in a planning system, buffer stocks and lead times are all fixed, and determine the outcome of the balance sheet (inventory on-hand). Instead, if the category manager questioned the lead time on material, they might be able to get to 50 – 75 days in a negotiated settlement, which would substantially improve the responsiveness of the planning system and increase velocity. In fact, Tom Linton notes that when he was the CSCO at Flex, he required all purchasing managers to slash their supplier lead times, as well as their planned internal assembly time. He did so on the basis of the assumption that humans were making subjective decisions on what lead times to load into the system. But if a machine were doing this work, it wouldn’t question the lead times. In this case, a human intervention is superior to a machine-based one.
A different example can be observed when one considers the situation of N95 masks in Covid. Because of the outbreak in Wuhan, the lead times for masks increased overnight. It likely took weeks for this information to filter through supply chains, and suddenly several weeks later planners came to realize that N95 masks were unavailable, and that the lead times had grown to nine months! A machine would have transmitted this information instantaneously through supply chain planning systems in seconds, and people could have acted much sooner to expedite or understand the problems with masks. This is a “positive vote for the machine”.
The point of this is that human context and decision-making has to be weighed off against one another. There is a real benefit to the social interaction of procurement, which leads to improved collaboration on difficult and complex problems. However, machines can be less judgmental, and render decisions without having to take pause, wait for their boss to come back from vacation, wait to get approval from a superior, or think about it for a few days. A new book called “Noise” reveals the incredible disparity in human decision-making, which can result in decisions which vary by as much as 40-75% when expert decision-makers are faced with the same set of information about a decision. This applies to multiple areas, including insurance premium adjusters, physicians reading radiology reports, courtroom judges handing out sentences, and many other areas.
The point here is that machines can often make better and more consistent and less biased decisions with less noise, when humans are faced with disparate decision outcomes even when presented with guidelines. On the other hand, in the book “Army of None”, a veteran notes that a machine may mistakenly identify a child or a passing dog as an enemy and kill it with a bomb strike, as it does not discern between moving objects and assumes they are always enemies if they are in the attack zone. The same logic applies to a planner who hears about lead time increases, and raises the minimum order quantity – the decision as to how much to raise it is better made by a machine, while the actual lead time negotiated may be better suited to a human decision-maker.
An important component that drives decision-making in an automated context is how you are measuring outcomes. Work activity is also typically influenced by how people are measured. If you are measured on cost – you will drive all of your activity to achieve that end, and a machine will as well. If you are measured on speed and velocity and reliability- than cost may be a tradeoff that has to be made. Recognizing these tradeoffs is at the very heart of the supply chain disruption dilemmas we find ourselves in today. And it’s time to face the music, as we have been too focused on cost for too long, and are paying the piper for those decisions today.